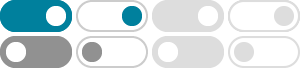
Understanding the Data Observability Diagram | Orchestra
Jul 19, 2024 · A data observability diagram illustrates the flow of data from its sources to its destinations, highlighting key stages such as extraction, transformation, and loading (ETL). It provides a comprehensive view of data lineage, data quality metrics, and potential points of failure within the data pipeline.
Data quality vs data observability: How they differ but work …
Mar 28, 2025 · Data quality ensures periodic validation, data observability is continuous. Data observability platforms continuously monitor your data’s health. In contrast, you might ensure data quality at specific points in time (like at the end of an ETL job or during a weekly data review). Observability provides an always-on view.
Data Observability vs Data Quality: Key Differences
Data observability tools track system performance metrics (latency, failures, anomalies), while data quality focuses on data accuracy metrics (completeness, consistency). Aligning them across tools requires standardization.
How to Integrate Data Quality into Data Pipelines - Diagrams …
Dec 13, 2024 · Read this guide to learn how to combine data quality, data observability and data pipelines to detect and prevent loading bad data.
Validating Your Data-Flow Diagrams: Ensuring Clarity and Accuracy
Oct 11, 2023 · However, the complexity of creating these diagrams necessitates a thorough validation process to ensure accuracy and clarity. Here, we’ll explore a set of questions and considerations to guide you in validating your DFDs effectively.
Data Observability and Data Quality: Improving Your Workflow ...
This article explores the concept of data observability and its significant role in improving data quality. We discuss essential practices and provide examples to help you start enhancing data quality using observability tools.
Data quality ensures that individual data points are accurate, complete, and consistent for their intended use. Data observability monitors the health of data pipelines in real-time, detecting issues with freshness, distribution, and other system-level factors that impact data quality.
DOF enables capture of data quality scores in a modelled exception database with business and technical context. External reporting integration for DQ dashboards, DQ Audit and Operational dashboards. The proposed Informatica Data Quality Reporting Framework will be built based on the following design principles.
Data Observability vs Data Quality: Understanding the …
Jul 16, 2024 · Data observability refers to the ability to fully understand and monitor the health and performance of data systems. It includes understanding data lineage, which helps track data flow, behavior, and characteristics. It involves monitoring and analyzing data flows, detecting anomalies, and gaining insights into the root causes of issues.
Data Pipeline Observability is Key to Data Quality - Datavolo
Apr 15, 2024 · By incorporating these features, Datavolo, powered by Apache NiFi, facilitates the establishment of robust data quality practices within data pipelines, ensuring that data is accurate, reliable, and meets the desired standards as it traverses the system.