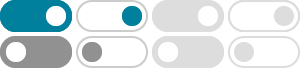
机器学习中的encoder,decoder和embedding都有什么区别? - 知乎
以transformer为例,最原始的结构有6层encoder,6层decoder,而embedding在encoder、decoder之前都会做一次,目的是将词转化为向量,也即word2vec,有许多方法能做到这一 …
Transformer的Decoder部分有什么改进思路吗? - 知乎
Mar 20, 2024 · 现在的问题是:Decoder层在预测下一个token时,是如何进行线性变换并在目标词汇表c中找出x4的。Decoder层在预测下一个token时,是如何进行线性变换并在目标词汇表c …
Decoder V - Netflix | Proximus Forum
Een tijdje geleden zijn we van de V5 decoder overgestapt naar de V6 decoder. Nu met deze nieuw decoder ondervinden we veel meer problemen dan met de oude. Als we via de decoder …
nieuwe decoder - Proximus Forum
Voor de decoder v6 hebben ze samengewerkt met Huawei. Ik kan niet meespreken over v6 omdat ikzelf maar v5 heb. Technicolor heeft gewoon de gehele productie lijn van scientific …
decoder-only和encoder-decoder transformer在应用时最大的区别 …
2、应用:Encoder-Decoder Transformer常用于序列到序列的任务,如机器翻译、对话生成等,可以处理输入序列和输出序列之间的对应关系。Decoder-Only Transformer主要用于生成式任 …
为什么现在的GPT模型都采用Decoder Only的架构? - 知乎
参数效率:与Encoder-Decoder架构相比,Decoder-only架构可以用相同的参数量构建更深的网络,从而提高模型的容量和性能,同时简化了模型复杂度。 灵活性 :Decoder-only架构在预训 …
为什么现在的LLM都是Decoder only的架构? - 知乎
Google在T5和UL2两篇论文中做了较为充分的对比实验,结果均体现出了Encoder-Decoder架构相比于Decoder-only的优势,但由于从LLM的角度看这两篇论文的模型尺度都还不算大,以及多 …
Afstandsbediening V7, mijn mening | Proximus Forum
Jun 10, 2024 · De afstandsbediening van de decoder V7 is moeilijker te gebruiken dan de vorige. De vorige was bijvoorbeeld handiger om voor- of achteruit te spoelen omdat je 4 standen voor …
神经网络都是encoder-decoder的结构吗? - 知乎
回到问题,神经网络都是encoder-decoder的结构吗? 当然不是,大部分神经网络都有encoder,而是不是有decoder主要取决于任务的目的是什么。事实上,如果按照编解码的含义来看,大部 …
Blauw led HD op V6 decoder - Proximus Forum
Uw decoder zal volledig worden geherinitialiseerd.). Ik had de indruk dat het amper iets langer heeft geduurd dan een herstart. Maar het resultaat was toch: terug 3 blauwe leds op een HD …