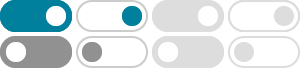
ML | Expectation-Maximization Algorithm - GeeksforGeeks
Feb 4, 2025 · The Expectation-Maximization (EM) algorithm is an iterative method used in unsupervised machine learning to estimate unknown parameters in statistical models. It helps find the best values for unknown parameters, especially when some data is missing or hidden.
Expectation-Maximization Algorithm on Python | by PRATEEK …
Sep 1, 2019 · Instead, we can use the expectation-maximization (EM) approach for finding the maximum likelihood estimates for the parameters θ. EM is a two-step iterative approach that starts from an...
Implementing Expectation-Maximisation Algorithm from Scratch with Python
Jan 19, 2022 · The Expectation-Maximisation (EM) Algorithm is a statistical Machine Learning method to find the maximum likelihood estimates of models with unknown latent variables. I am sure that that sentence will make no sense to some of you.
K-Means and EM Algorithm in Python - VTUPulse.com
Apply EM algorithm to cluster a set of data stored in a .CSV file. Use the same data set for clustering using the k-Means algorithm. Compare the results of these two algorithms and comment on the quality of clustering.
Expectation Maximizatio (EM) Algorithm — Computational …
So the basic idea behind Expectation Maximization (EM) is simply to start with a guess for θ θ, then calculate z z, then update θ θ using this new value for z z, and repeat till convergence. The derivation below shows why the EM algorithm using this “alternating” updates actually works.
GitHub - mr-easy/GMM-EM-Python: Python implementation of EM algorithm …
Python implementation of Expectation-Maximization algorithm (EM) for Gaussian Mixture Model (GMM). Code for GMM is in GMM.py. It's very well documented on how to use it on your data. For an example and visualization for 2D set of points, see the notebook EM_for_2D_GMM.ipynb. class GMM: Defines a model with known number of clusters and dimensions.
Exploring the EM Algorithm Python Package: Concepts, Usage, …
Jan 29, 2025 · The Expectation-Maximization (EM) algorithm is a powerful iterative method used in statistics and machine learning for maximum likelihood estimation (MLE) in the presence of latent variables. Python offers several packages that implement the EM algorithm, making it accessible for data scientists and researchers to solve complex problems.
Expectation-maximization algorithm, explained · Xiaozhou's Notes
Oct 20, 2020 · Expectation-maximization algorithm, explained 20 Oct 2020. A comprehensive guide to the EM algorithm with intuitions, examples, Python implementation, and maths. Yes! Let’s talk about the expectation-maximization algorithm (EM, for short).
Expectation Maximization Algorithm (EM) Implement in Python …
Feb 1, 2021 · In this article, we explored how to train Gaussian Mixture Models with the Expectation-Maximization Algorithm and implemented it in Python to solve unsupervised and semi-supervised learning problems. EM is a very useful method to find the maximum likelihood when the model depends on latent variables and therefore is frequently used in machine ...
A Gentle Introduction to Expectation-Maximization (EM Algorithm)
Aug 28, 2020 · Maximum likelihood estimation is an approach to density estimation for a dataset by searching across probability distributions and their parameters.
- Some results have been removed