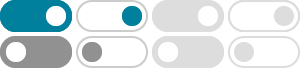
Machine Learning : Handling Dataset having Multiple Features
Well, this covers gist about handling multivariate dataset, making classifications, doing predictions and also simplifying into lower dimensions for data compression and better visualization purpose.
MGLNN: Semi-supervised learning via Multiple Graph Cooperative Learning …
Sep 1, 2022 · In this paper, we propose a novel learning framework, called Multiple Graph Learning Neural Networks (MGLNN), for multiple graph learning and multi-view semi-supervised classification.
Graph neural networks for multi-view learning: a taxonomic review
Oct 21, 2024 · The single-channel learning approach employs a shared-weight graph neural network to process multiple adjacency graphs along with their corresponding feature matrices, extracting representations from different perspectives.
Mosaic of Modalities: A Comprehensive Benchmark for Multimodal Graph …
Jun 24, 2024 · To support advancements in this emerging field, we provide an extensive empirical study on various graph learning frameworks when presented with features from multiple modalities, particularly emphasizing the impact of visual information.
Enhancing graph structure learning through multiple features and graphs …
Apr 1, 2025 · To cope with these issues, this paper introduces MFGSL (multiple feature graph structure learning), a novel approach that simultaneously learns and merges multiple graph structures and features to learn an informative and comprehensive graph structure.
An adaptive multi-graph neural network with multimodal feature …
Nov 18, 2024 · We propose a multimodal depression detection framework called EMO-GCN, which uses multiple graph convolutional networks to extract structural features from EEG signals and acoustic features...
[2504.17749] MSGCN: Multiplex Spatial Graph Convolution …
4 days ago · Graph Neural Networks (GNNs) have been widely used for various learning tasks, ranging from node classification to link prediction. They have demonstrated excellent performance in multiple domains involving graph-structured data. However, an important category of learning tasks, namely link weight prediction, has received less emphasis due to its increased complexity compared to binary link ...
Collaborative Multi-Agent Reinforcement Learning for Automated Feature …
4 days ago · To address the limitations, we propose TCTO, a collaborative multi-agent reinforcement learning framework that automates feature engineering through graph-driven path optimization. The framework's core innovation lies in an evolving interaction graph that models features as nodes and transformations as edges.
machine learning - What graph structures can describe a dataset …
Dec 16, 2024 · Instead if you want to analyze feature relationships for feature selection/ranking or multicollinearity analysis or dimensionality reduction, then a feature correlation graph is most suitable where nodes represent features (not data points) and edge weights are based on statistical measures like Pearson correlation or Spearman's rank correlation...
Rethinking Graph Prompts: Unraveling the Power of Data …
1 day ago · Unlike traditional fine-tuning, graph prompts rewrite graph structures and features through components like prompt tokens and insertion patterns, improving flexibility and efficiency. Applications in IoT, drug discovery, fraud detection, and personalized learning demonstrate their potential to dynamically adapt graph data.
- Some results have been removed