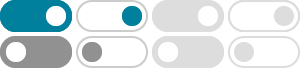
tensorflow - Parallelization strategies for deep learning - Stack Overflow
May 30, 2020 · In general, there are two strategies of parallelizing model training: data parallelism and model parallelism. 1. Data parallelism. This strategy splits training data into N partitions, each of which will be trained on different “devices” (different CPU cores, GPUs, or even machines).
Distributed training with TensorFlow
Oct 25, 2024 · tf.distribute.Strategy is a TensorFlow API to distribute training across multiple GPUs, multiple machines, or TPUs. Using this API, you can distribute your existing models and training code with minimal code changes. Provide good performance out of the box. Easy switching between strategies.
Multi-GPU and distributed training | TensorFlow Core
Specifically, this guide teaches you how to use the tf.distribute API to train Keras models on multiple GPUs, with minimal changes to your code, in the following two setups: On multiple GPUs (typically 2 to 8) installed on a single machine (single host, multi-device training).
Distributed Data Parallelism in TensorFlow - Scaler
Dec 7, 2023 · To implement distributed data parallelism in TensorFlow, developers need to divide the model and data, and assign them to different devices or machines. This can be done using TensorFlow's built-in distribution strategies, such as tf.distribute.MirroredStrategy or tf.distribute.experimental.MultiWorkerMirroredStrategy.
TensorFlow GPU Unleashing the Power of Parallel Computing
Jan 11, 2024 · TensorFlow can accelerate training and inference processes by harnessing the parallel computing power of GPUs, reducing the time required to build and deploy models. One of the key advantages of TensorFlow GPU is its ability to handle large-scale datasets.
TensorFlow - Multi GPU Computation | Sebastian Schöner
Dec 15, 2017 · It is an introduction to multi GPU computation in TensorFlow written for some colleagues in November 2017. The version of TensorFlow that this tutorial is targeting is v1.3. Making multi GPU training of models easier is, as I understand, one of the priorities of the TensorFlow development team.
Boosting Performance: A Guide to Parallel Processing in TensorFlow …
Apr 28, 2024 · Parallel processing is a powerful technique for accelerating machine learning model training in TensorFlow. By leveraging the tf.distribute APIs, you can distribute your computation across multiple devices, reducing training …
How to Use Distributed TensorFlow to Split Your TensorFlow Graph ...
Jun 14, 2018 · In model parallelism, we simply wish to divide our graph between multiple nodes because our graph is too large to be stored on a single GPU. As a result, I put together a basic example that...
Harnessing the Power of Parallelism for Faster Deep Learning …
Apr 28, 2023 · TensorFlow, a popular deep learning framework, provides various strategies for implementing parallelism and distributed training to speed up the learning process. In this blog post, we will...
Does TensorFlow automatically parallelize graph computations?
The reason is that TensorFlow does not supply an automatic mechanism for distributing a graph over multiple execution units, it is assumed that the developers do that themselves. However, it obviously keeps track of all the dependencies in the graph so that if you do it manually, it will make sure things are computed in the right order.
- Some results have been removed